Project flow#
LaminDB allows tracking data flow on the entire project level.
Here, we walk through exemplified app uploads, pipelines & notebooks following Schmidt et al., 2022.
A CRISPR screen reading out a phenotypic endpoint on T cells is paired with scRNA-seq to generate insights into IFN-γ production.
These insights get linked back to the original data through the steps taken in the project to provide context for interpretation & future decision making.
More specifically: Why should I care about data flow?
Data flow tracks data sources & transformations to trace biological insights, verify experimental outcomes, meet regulatory standards, increase the robustness of research and optimize the feedback loop of team-wide learning iterations.
While tracking data flow is easier when it’s governed by deterministic pipelines, it becomes hard when it’s governed by interactive human-driven analyses.
LaminDB interfaces workflow mangers for the former and embraces the latter.
Setup#
Init a test instance:
!lamin init --storage ./mydata
Show code cell output
✅ saved: User(uid='DzTjkKse', handle='testuser1', name='Test User1', updated_at=2023-11-15 15:54:09 UTC)
✅ saved: Storage(uid='yLwE278k', root='/home/runner/work/lamin-usecases/lamin-usecases/docs/mydata', type='local', updated_at=2023-11-15 15:54:09 UTC, created_by_id=1)
💡 loaded instance: testuser1/mydata
💡 did not register local instance on hub
Import lamindb:
import lamindb as ln
from IPython.display import Image, display
💡 lamindb instance: testuser1/mydata
Steps#
In the following, we walk through exemplified steps covering different types of transforms (Transform
).
Note
The full notebooks are in this repository.
App upload of phenotypic data
#
Register data through app upload from wetlab by testuser1
:
# This function mimics the upload of files via the UI
# In reality, you simply drag and drop files into the UI
def run_upload_crispra_result_app():
ln.setup.login("testuser1")
transform = ln.Transform(name="Upload GWS CRISPRa result", type="app")
ln.track(transform)
output_path = ln.dev.datasets.schmidt22_crispra_gws_IFNG(ln.settings.storage)
output_file = ln.File(output_path, description="Raw data of schmidt22 crispra GWS")
output_file.save()
run_upload_crispra_result_app()
Show code cell output
💡 saved: Transform(uid='Yq4DwAZOUX6Ncg', name='Upload GWS CRISPRa result', type='app', updated_at=2023-11-15 15:54:11 UTC, created_by_id=1)
💡 saved: Run(uid='EJdfIGGiBGPJlz5mnFBy', run_at=2023-11-15 15:54:11 UTC, transform_id=1, created_by_id=1)
Hit identification in notebook
#
Access, transform & register data in drylab by testuser2
:
def run_hit_identification_notebook():
# log in as another user
ln.setup.login("testuser2")
# create a new transform to mimic a new notebook (in reality you just run ln.track() in a notebook)
transform = ln.Transform(name="GWS CRIPSRa analysis", type="notebook")
ln.track(transform)
# access the upload file
input_file = ln.File.filter(key="schmidt22-crispra-gws-IFNG.csv").one()
# identify hits
input_df = input_file.load().set_index("id")
output_df = input_df[input_df["pos|fdr"] < 0.01].copy()
# register hits in output file
ln.File(output_df, description="hits from schmidt22 crispra GWS").save()
run_hit_identification_notebook()
Show code cell output
💡 saved: Transform(uid='WQUdDk750bfjEC', name='GWS CRIPSRa analysis', type='notebook', updated_at=2023-11-15 15:54:12 UTC, created_by_id=1)
💡 saved: Run(uid='lkPx9c5petcohWzZJbZg', run_at=2023-11-15 15:54:12 UTC, transform_id=2, created_by_id=1)
Inspect data flow:
file = ln.File.filter(description="hits from schmidt22 crispra GWS").one()
file.view_flow()
Sequencer upload
#
Upload files from sequencer:
def run_upload_from_sequencer_pipeline():
ln.setup.login("testuser1")
# create a pipeline transform
ln.track(ln.Transform(name="Chromium 10x upload", type="pipeline"))
# register output files of the sequencer
upload_dir = ln.dev.datasets.dir_scrnaseq_cellranger(
"perturbseq", basedir=ln.settings.storage, output_only=False
)
ln.File(upload_dir.parent / "fastq/perturbseq_R1_001.fastq.gz").save()
ln.File(upload_dir.parent / "fastq/perturbseq_R2_001.fastq.gz").save()
run_upload_from_sequencer_pipeline()
Show code cell output
💡 saved: Transform(uid='SOn4EXtXNEZe2B', name='Chromium 10x upload', type='pipeline', updated_at=2023-11-15 15:54:13 UTC, created_by_id=1)
💡 saved: Run(uid='OUm4qaYxMxiNTUcXNGSR', run_at=2023-11-15 15:54:13 UTC, transform_id=3, created_by_id=1)
❗ file has more than one suffix (path.suffixes), inferring: '.fastq.gz'
❗ file has more than one suffix (path.suffixes), inferring: '.fastq.gz'
scRNA-seq bioinformatics pipeline
#
Process uploaded files using a script or workflow manager: Pipelines and obtain 3 output files in a directory filtered_feature_bc_matrix/
:
def run_scrna_analysis_pipeline():
ln.setup.login("testuser2")
transform = ln.Transform(name="Cell Ranger", version="7.2.0", type="pipeline")
ln.track(transform)
# access uploaded files as inputs for the pipeline
input_files = ln.File.filter(key__startswith="fastq/perturbseq").all()
input_paths = [file.stage() for file in input_files]
# register output files
output_files = ln.File.from_dir("./mydata/perturbseq/filtered_feature_bc_matrix/")
ln.save(output_files)
# Post-process these 3 files
transform = ln.Transform(
name="Postprocess Cell Ranger", version="2.0", type="pipeline"
)
ln.track(transform)
input_files = [f.stage() for f in output_files]
output_path = ln.dev.datasets.schmidt22_perturbseq(basedir=ln.settings.storage)
output_file = ln.File(output_path, description="perturbseq counts")
output_file.save()
run_scrna_analysis_pipeline()
Show code cell output
💡 saved: Transform(uid='lUjNhRN4MaozX1', name='Cell Ranger', version='7.2.0', type='pipeline', updated_at=2023-11-15 15:54:14 UTC, created_by_id=1)
💡 saved: Run(uid='yfvSXgkylBAL0LQAYAiq', run_at=2023-11-15 15:54:14 UTC, transform_id=4, created_by_id=1)
❗ file has more than one suffix (path.suffixes), inferring: '.mtx.gz'
❗ file has more than one suffix (path.suffixes), inferring: '.tsv.gz'
❗ file has more than one suffix (path.suffixes), inferring: '.tsv.gz'
💡 saved: Transform(uid='8uk9bafLthkGsd', name='Postprocess Cell Ranger', version='2.0', type='pipeline', updated_at=2023-11-15 15:54:14 UTC, created_by_id=1)
💡 saved: Run(uid='Hr8JyH13JceNTdpi8KUN', run_at=2023-11-15 15:54:14 UTC, transform_id=5, created_by_id=1)
Inspect data flow:
output_file = ln.File.filter(description="perturbseq counts").one()
output_file.view_flow()
Integrate scRNA-seq & phenotypic data
#
Integrate data in a notebook:
def run_integrated_analysis_notebook():
import scanpy as sc
# create a new transform to mimic a new notebook (in reality you just run ln.track() in a notebook)
transform = ln.Transform(
name="Perform single cell analysis, integrate with CRISPRa screen",
type="notebook",
)
ln.track(transform)
# access the output files of bfx pipeline and previous analysis
file_ps = ln.File.filter(description__icontains="perturbseq").one()
adata = file_ps.load()
file_hits = ln.File.filter(description="hits from schmidt22 crispra GWS").one()
screen_hits = file_hits.load()
# perform analysis and register output plot files
sc.tl.score_genes(adata, adata.var_names.intersection(screen_hits.index).tolist())
filesuffix = "_fig1_score-wgs-hits.png"
sc.pl.umap(adata, color="score", show=False, save=filesuffix)
filepath = f"figures/umap{filesuffix}"
file = ln.File(filepath, key=filepath)
file.save()
filesuffix = "fig2_score-wgs-hits-per-cluster.png"
sc.pl.matrixplot(
adata, groupby="cluster_name", var_names=["score"], show=False, save=filesuffix
)
filepath = f"figures/matrixplot_{filesuffix}"
file = ln.File(filepath, key=filepath)
file.save()
run_integrated_analysis_notebook()
Show code cell output
💡 saved: Transform(uid='i2TkopQ2PkkD6g', name='Perform single cell analysis, integrate with CRISPRa screen', type='notebook', updated_at=2023-11-15 15:54:16 UTC, created_by_id=1)
💡 saved: Run(uid='ZZ4wJtaPhYZXH628lgYF', run_at=2023-11-15 15:54:16 UTC, transform_id=6, created_by_id=1)
WARNING: saving figure to file figures/umap_fig1_score-wgs-hits.png
WARNING: saving figure to file figures/matrixplot_fig2_score-wgs-hits-per-cluster.png
Review results#
Let’s load one of the plots:
# track the current notebook as transform
ln.track()
file = ln.File.filter(key__contains="figures/matrixplot").one()
file.stage()
Show code cell output
💡 notebook imports: ipython==8.17.2 lamindb==0.61.0 scanpy==1.9.6
💡 saved: Transform(uid='1LCd8kco9lZUz8', name='Project flow', short_name='project-flow', version='0', type=notebook, updated_at=2023-11-15 15:54:17 UTC, created_by_id=1)
💡 saved: Run(uid='S0ESDDjVgXvnBbS1F80M', run_at=2023-11-15 15:54:17 UTC, transform_id=7, created_by_id=1)
PosixUPath('/home/runner/work/lamin-usecases/lamin-usecases/docs/mydata/.lamindb/Jg739VeQIBQMx1bnhV3F.png')
display(Image(filename=file.path))
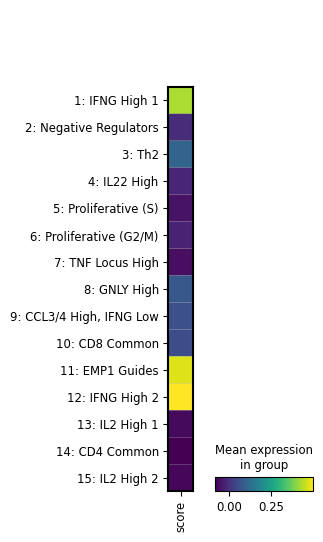
We see that the image file is tracked as an input of the current notebook. The input is highlighted, the notebook follows at the bottom:
file.view_flow()
Alternatively, we can also look at the sequence of transforms:
transform = ln.Transform.search("Bird's eye view", return_queryset=True).first()
transform.parents.df()
uid | name | short_name | version | type | latest_report_id | source_file_id | reference | reference_type | initial_version_id | updated_at | created_by_id | |
---|---|---|---|---|---|---|---|---|---|---|---|---|
id | ||||||||||||
4 | lUjNhRN4MaozX1 | Cell Ranger | None | 7.2.0 | pipeline | None | None | None | None | None | 2023-11-15 15:54:14.381672+00:00 | 1 |
transform.view_parents()
Understand runs#
We tracked pipeline and notebook runs through run_context
, which stores a Transform
and a Run
record as a global context.
File
objects are the inputs and outputs of runs.
What if I don’t want a global context?
Sometimes, we don’t want to create a global run context but manually pass a run when creating a file:
run = ln.Run(transform=transform)
ln.File(filepath, run=run)
When does a file appear as a run input?
When accessing a file via stage()
, load()
or backed()
, two things happen:
The current run gets added to
file.input_of
The transform of that file gets added as a parent of the current transform
You can then switch off auto-tracking of run inputs if you set ln.settings.track_run_inputs = False
: Can I disable tracking run inputs?
You can also track run inputs on a case by case basis via is_run_input=True
, e.g., here:
file.load(is_run_input=True)
Query by provenance#
We can query or search for the notebook that created the file:
transform = ln.Transform.search("GWS CRIPSRa analysis", return_queryset=True).first()
And then find all the files created by that notebook:
ln.File.filter(transform=transform).df()
uid | storage_id | key | suffix | accessor | description | version | size | hash | hash_type | transform_id | run_id | initial_version_id | visibility | key_is_virtual | updated_at | created_by_id | |
---|---|---|---|---|---|---|---|---|---|---|---|---|---|---|---|---|---|
id | |||||||||||||||||
2 | 2jM5FLweMJ1bpCkJGXMq | 1 | None | .parquet | DataFrame | hits from schmidt22 crispra GWS | None | 18368 | fjvT1POciz7QFCK6Wzaflw | md5 | 2 | 2 | None | 0 | True | 2023-11-15 15:54:12.902871+00:00 | 1 |
Which transform ingested a given file?
file = ln.File.filter().first()
file.transform
Transform(uid='Yq4DwAZOUX6Ncg', name='Upload GWS CRISPRa result', type='app', updated_at=2023-11-15 15:54:11 UTC, created_by_id=1)
And which user?
file.created_by
User(uid='DzTjkKse', handle='testuser1', name='Test User1', updated_at=2023-11-15 15:54:13 UTC)
Which transforms were created by a given user?
users = ln.User.lookup()
ln.Transform.filter(created_by=users.testuser2).df()
uid | name | short_name | version | type | reference | reference_type | updated_at | latest_report_id | source_file_id | initial_version_id | created_by_id | |
---|---|---|---|---|---|---|---|---|---|---|---|---|
id |
Which notebooks were created by a given user?
ln.Transform.filter(created_by=users.testuser2, type="notebook").df()
uid | name | short_name | version | type | reference | reference_type | updated_at | latest_report_id | source_file_id | initial_version_id | created_by_id | |
---|---|---|---|---|---|---|---|---|---|---|---|---|
id |
We can also view all recent additions to the entire database:
ln.view()
Show code cell output
File
uid | storage_id | key | suffix | accessor | description | version | size | hash | hash_type | transform_id | run_id | initial_version_id | visibility | key_is_virtual | updated_at | created_by_id | |
---|---|---|---|---|---|---|---|---|---|---|---|---|---|---|---|---|---|
id | |||||||||||||||||
10 | Jg739VeQIBQMx1bnhV3F | 1 | figures/matrixplot_fig2_score-wgs-hits-per-clu... | .png | None | None | None | 28814 | ND6ylOuI7Any3XCTJ9L4Og | md5 | 6 | 6 | None | 0 | True | 2023-11-15 15:54:16.681473+00:00 | 1 |
9 | qQo7URh6F1g0bKCo1y5Z | 1 | figures/umap_fig1_score-wgs-hits.png | .png | None | None | None | 118999 | d-7QvMjLXT10P6SlzmFqXA | md5 | 6 | 6 | None | 0 | True | 2023-11-15 15:54:16.429047+00:00 | 1 |
8 | YaoV7UFg8zYwdaPqJdm9 | 1 | schmidt22_perturbseq.h5ad | .h5ad | AnnData | perturbseq counts | None | 20659936 | la7EvqEUMDlug9-rpw-udA | md5 | 5 | 5 | None | 0 | False | 2023-11-15 15:54:14.959283+00:00 | 1 |
7 | Z9hK64oYeiMECvEvKUEM | 1 | perturbseq/filtered_feature_bc_matrix/features... | .tsv.gz | None | None | None | 6 | GzYzVcW-t6d9nnO0BhWk4A | md5 | 4 | 4 | None | 0 | False | 2023-11-15 15:54:14.422537+00:00 | 1 |
6 | 3rib4xhvPQUb6hkGJ5j3 | 1 | perturbseq/filtered_feature_bc_matrix/barcodes... | .tsv.gz | None | None | None | 6 | lMcD9sOeh0cm2AArh5Whnw | md5 | 4 | 4 | None | 0 | False | 2023-11-15 15:54:14.422025+00:00 | 1 |
5 | 6vOVhJmas9UgeGQEZ5yH | 1 | perturbseq/filtered_feature_bc_matrix/matrix.m... | .mtx.gz | None | None | None | 6 | rq8URjAUj841GuK_qDBRdA | md5 | 4 | 4 | None | 0 | False | 2023-11-15 15:54:14.421406+00:00 | 1 |
4 | TaVNCTws08dWgmHcqNbp | 1 | fastq/perturbseq_R2_001.fastq.gz | .fastq.gz | None | None | None | 6 | hnvH0hdRUIbTf6pHtFBXlw | md5 | 3 | 3 | None | 0 | False | 2023-11-15 15:54:13.672682+00:00 | 1 |
Run
uid | transform_id | run_at | created_by_id | report_id | is_consecutive | reference | reference_type | |
---|---|---|---|---|---|---|---|---|
id | ||||||||
1 | EJdfIGGiBGPJlz5mnFBy | 1 | 2023-11-15 15:54:11.891456+00:00 | 1 | None | None | None | None |
2 | lkPx9c5petcohWzZJbZg | 2 | 2023-11-15 15:54:12.827024+00:00 | 1 | None | None | None | None |
3 | OUm4qaYxMxiNTUcXNGSR | 3 | 2023-11-15 15:54:13.655273+00:00 | 1 | None | None | None | None |
4 | yfvSXgkylBAL0LQAYAiq | 4 | 2023-11-15 15:54:14.386595+00:00 | 1 | None | None | None | None |
5 | Hr8JyH13JceNTdpi8KUN | 5 | 2023-11-15 15:54:14.437221+00:00 | 1 | None | None | None | None |
6 | ZZ4wJtaPhYZXH628lgYF | 6 | 2023-11-15 15:54:16.136440+00:00 | 1 | None | None | None | None |
7 | S0ESDDjVgXvnBbS1F80M | 7 | 2023-11-15 15:54:17.167579+00:00 | 1 | None | None | None | None |
Storage
uid | root | type | region | updated_at | created_by_id | |
---|---|---|---|---|---|---|
id | ||||||
1 | yLwE278k | /home/runner/work/lamin-usecases/lamin-usecase... | local | None | 2023-11-15 15:54:09.994236+00:00 | 1 |
Transform
uid | name | short_name | version | type | latest_report_id | source_file_id | reference | reference_type | initial_version_id | updated_at | created_by_id | |
---|---|---|---|---|---|---|---|---|---|---|---|---|
id | ||||||||||||
7 | 1LCd8kco9lZUz8 | Project flow | project-flow | 0 | notebook | None | None | None | None | None | 2023-11-15 15:54:17.162725+00:00 | 1 |
6 | i2TkopQ2PkkD6g | Perform single cell analysis, integrate with C... | None | None | notebook | None | None | None | None | None | 2023-11-15 15:54:16.129867+00:00 | 1 |
5 | 8uk9bafLthkGsd | Postprocess Cell Ranger | None | 2.0 | pipeline | None | None | None | None | None | 2023-11-15 15:54:14.433456+00:00 | 1 |
4 | lUjNhRN4MaozX1 | Cell Ranger | None | 7.2.0 | pipeline | None | None | None | None | None | 2023-11-15 15:54:14.381672+00:00 | 1 |
3 | SOn4EXtXNEZe2B | Chromium 10x upload | None | None | pipeline | None | None | None | None | None | 2023-11-15 15:54:13.651248+00:00 | 1 |
2 | WQUdDk750bfjEC | GWS CRIPSRa analysis | None | None | notebook | None | None | None | None | None | 2023-11-15 15:54:12.821082+00:00 | 1 |
1 | Yq4DwAZOUX6Ncg | Upload GWS CRISPRa result | None | None | app | None | None | None | None | None | 2023-11-15 15:54:11.885779+00:00 | 1 |
User
uid | handle | name | updated_at | |
---|---|---|---|---|
id | ||||
2 | bKeW4T6E | testuser2 | Test User2 | 2023-11-15 15:54:14.370930+00:00 |
1 | DzTjkKse | testuser1 | Test User1 | 2023-11-15 15:54:13.639512+00:00 |
Show code cell content
!lamin login testuser1
!lamin delete --force mydata
!rm -r ./mydata
✅ logged in with email testuser1@lamin.ai (uid: DzTjkKse)
💡 deleting instance testuser1/mydata
✅ deleted instance settings file: /home/runner/.lamin/instance--testuser1--mydata.env
✅ instance cache deleted
✅ deleted '.lndb' sqlite file
❗ consider manually deleting your stored data: /home/runner/work/lamin-usecases/lamin-usecases/docs/mydata